Town Change
EXPERIMENT 2
From a city to a bucolic country
It tests the generalization and adaptation performances of the semantic segmentation networks when changing completely the environment, moving from a city to a countryside. The distance between the source and target scenario is high so, as a consequence, the task is difficult. The task is even more challenging due to the rain condition.
Source Scenario:
- Environment: Town 01
- Weather and illumination condition: Hard Rain Noon
- Viewpoint: Audi TT
- Source train set size: 10008
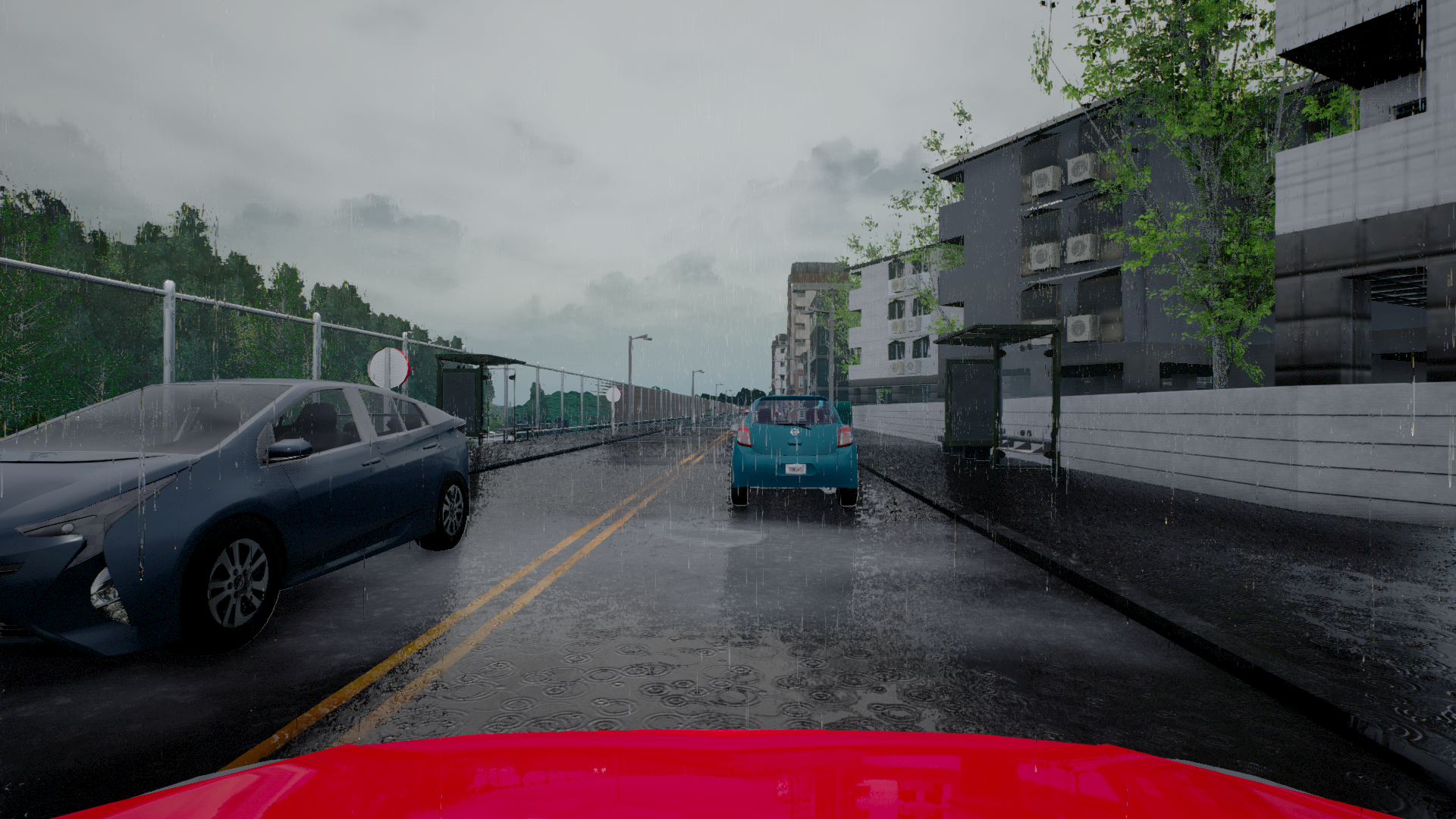
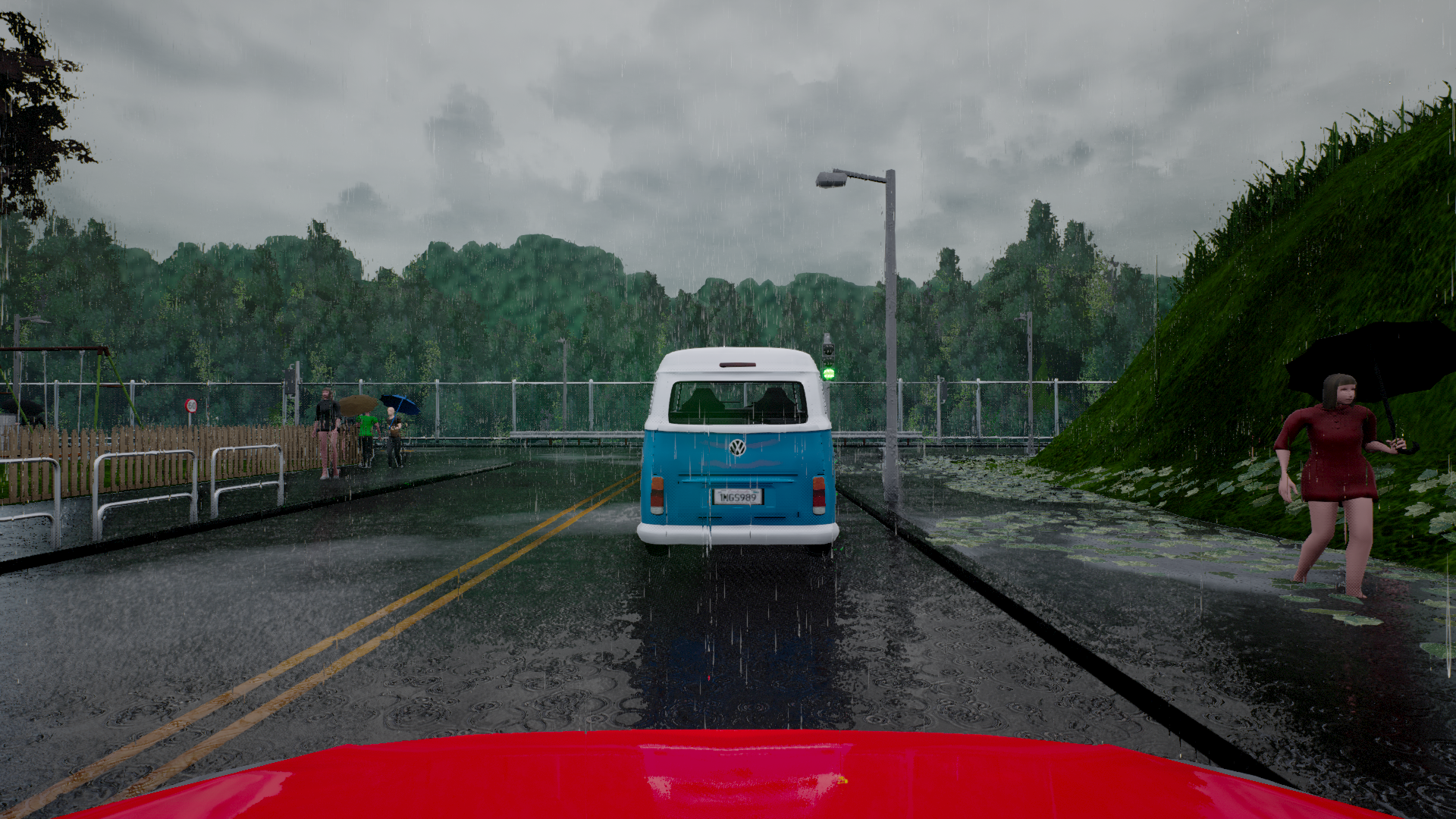
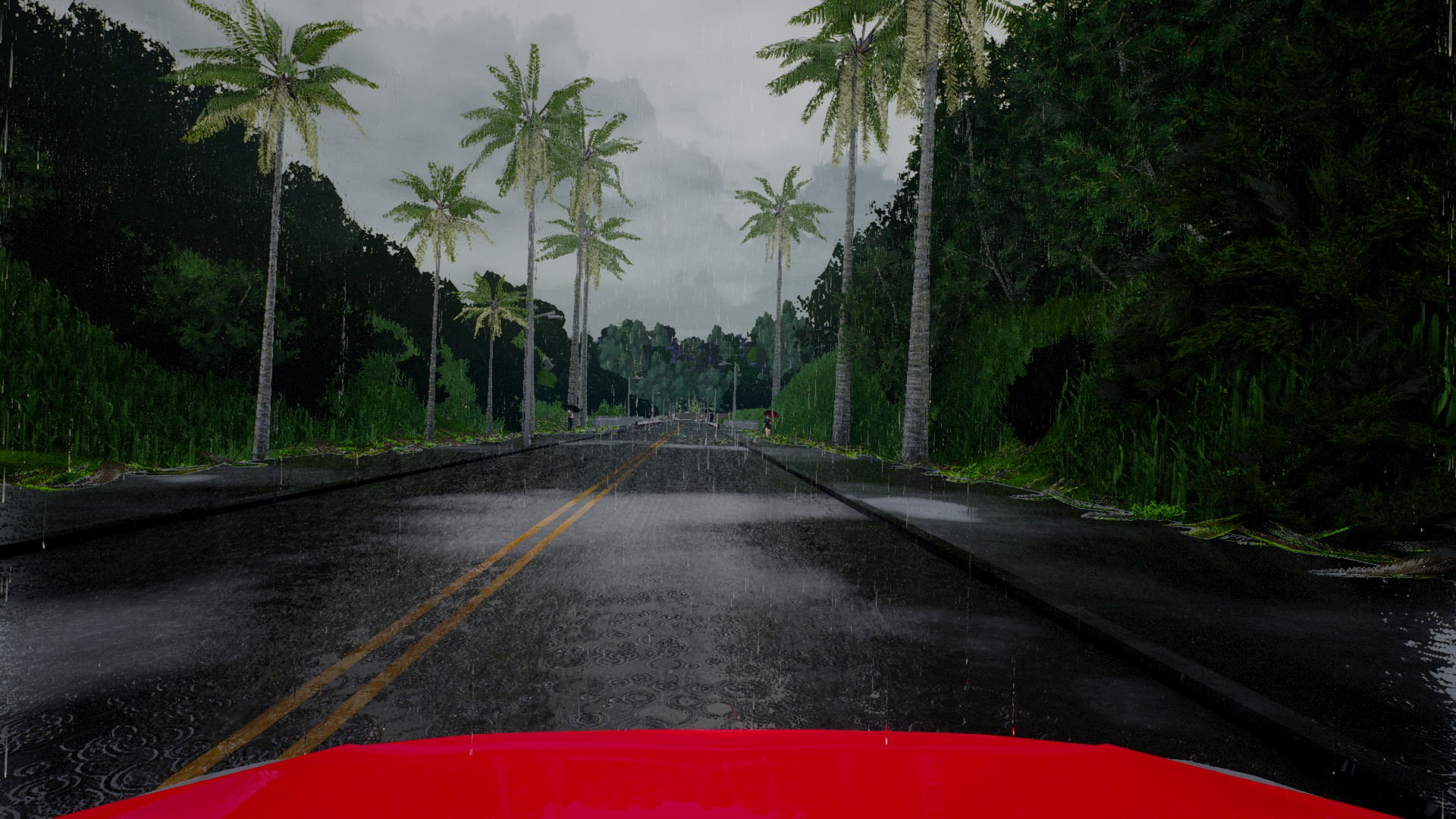
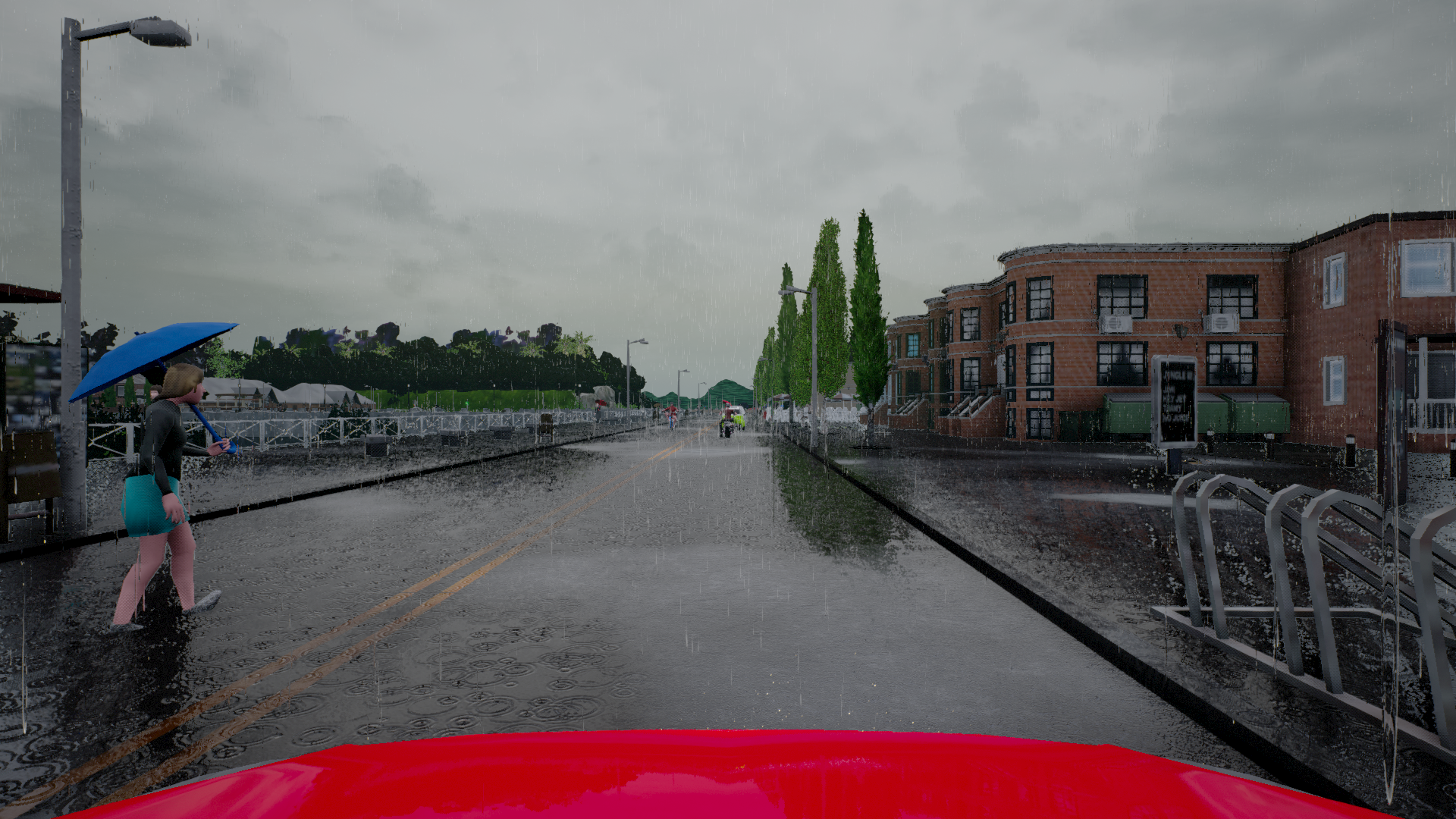
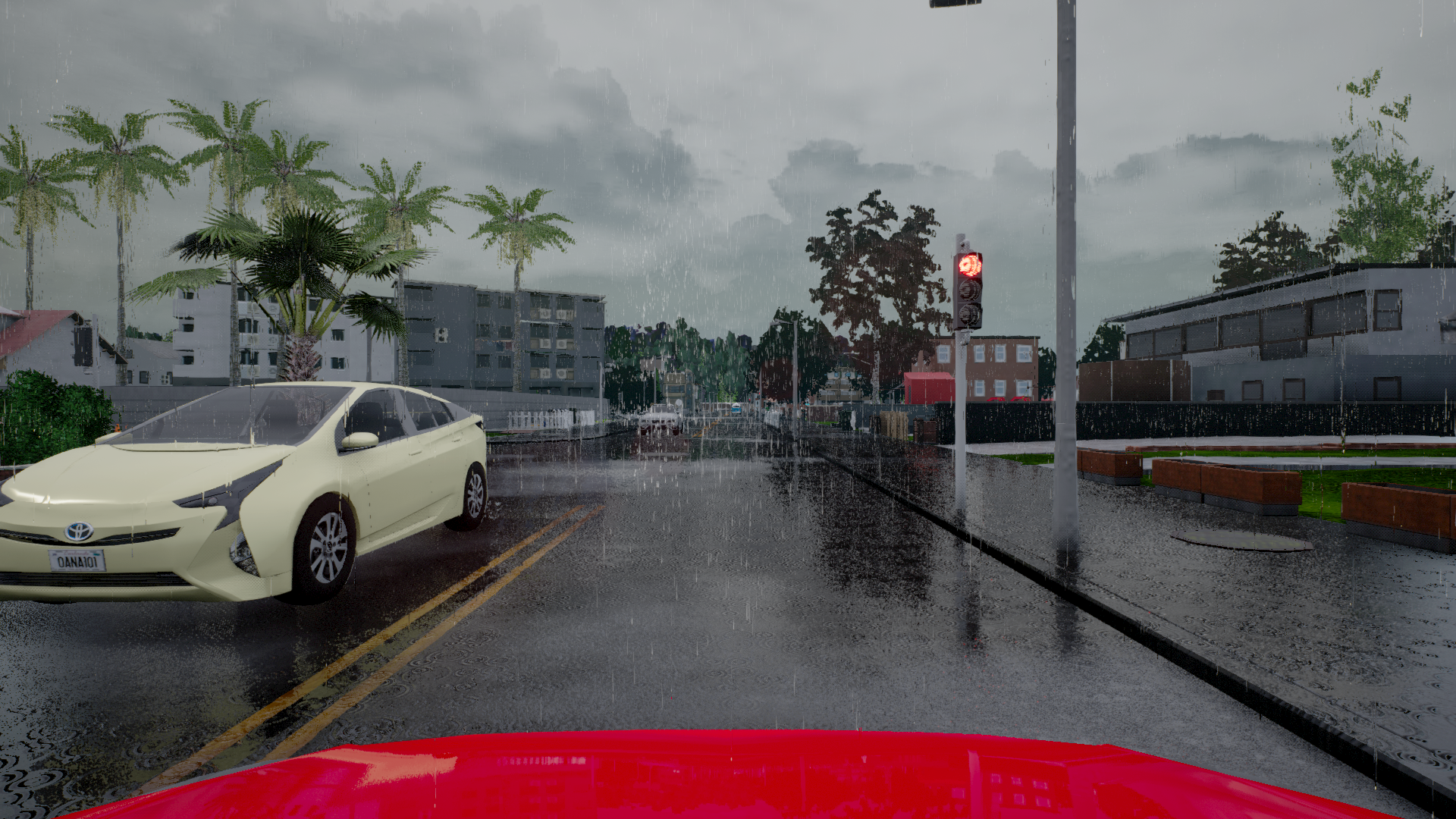
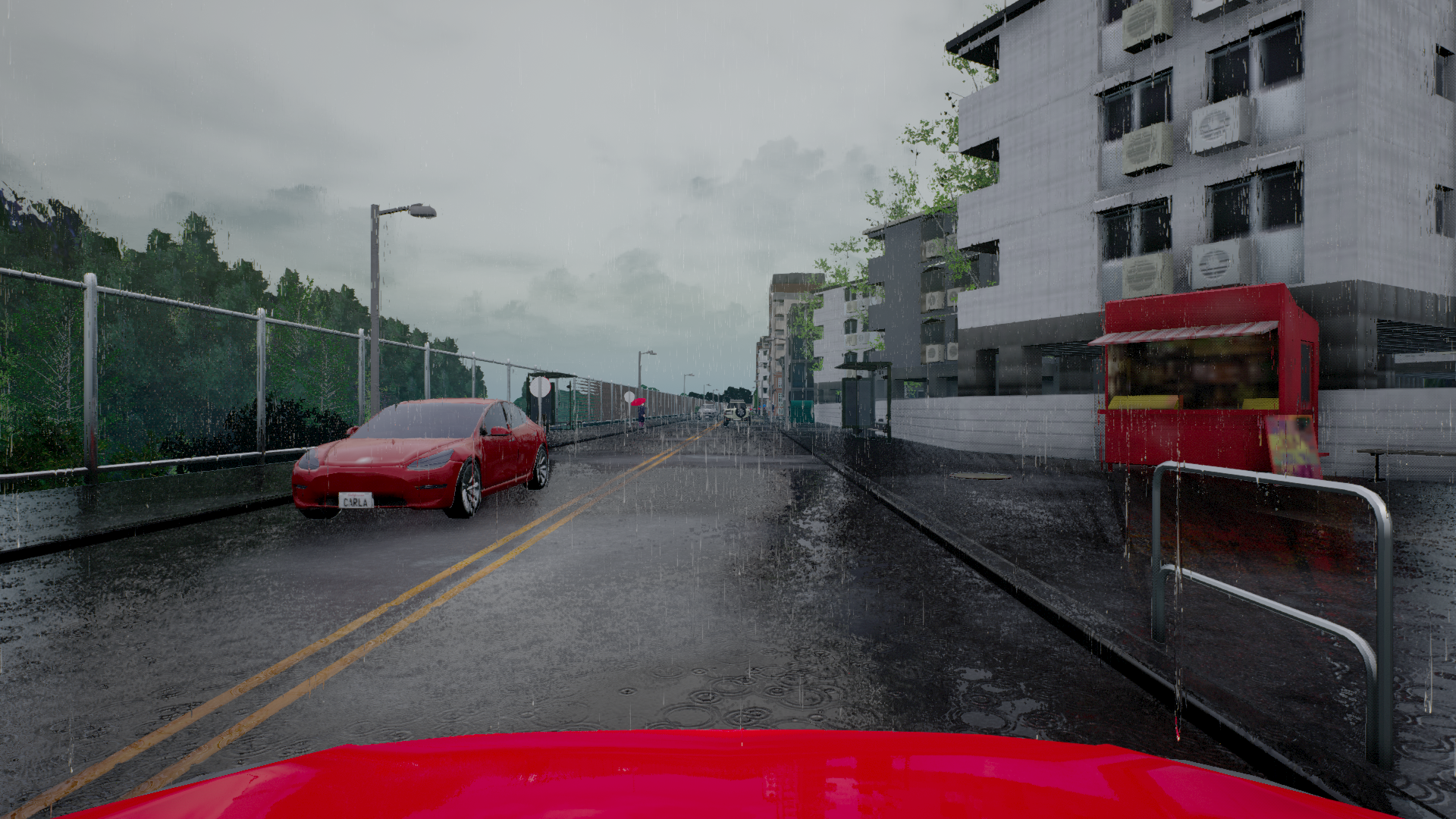
Target/Test Scenario:
- Environment: Town 07
- Weather and illumination condition: Hard Rain Noon
- Viewpoint: Audi TT
- Target train set size (only with DA): 10008
- Test size: 1672
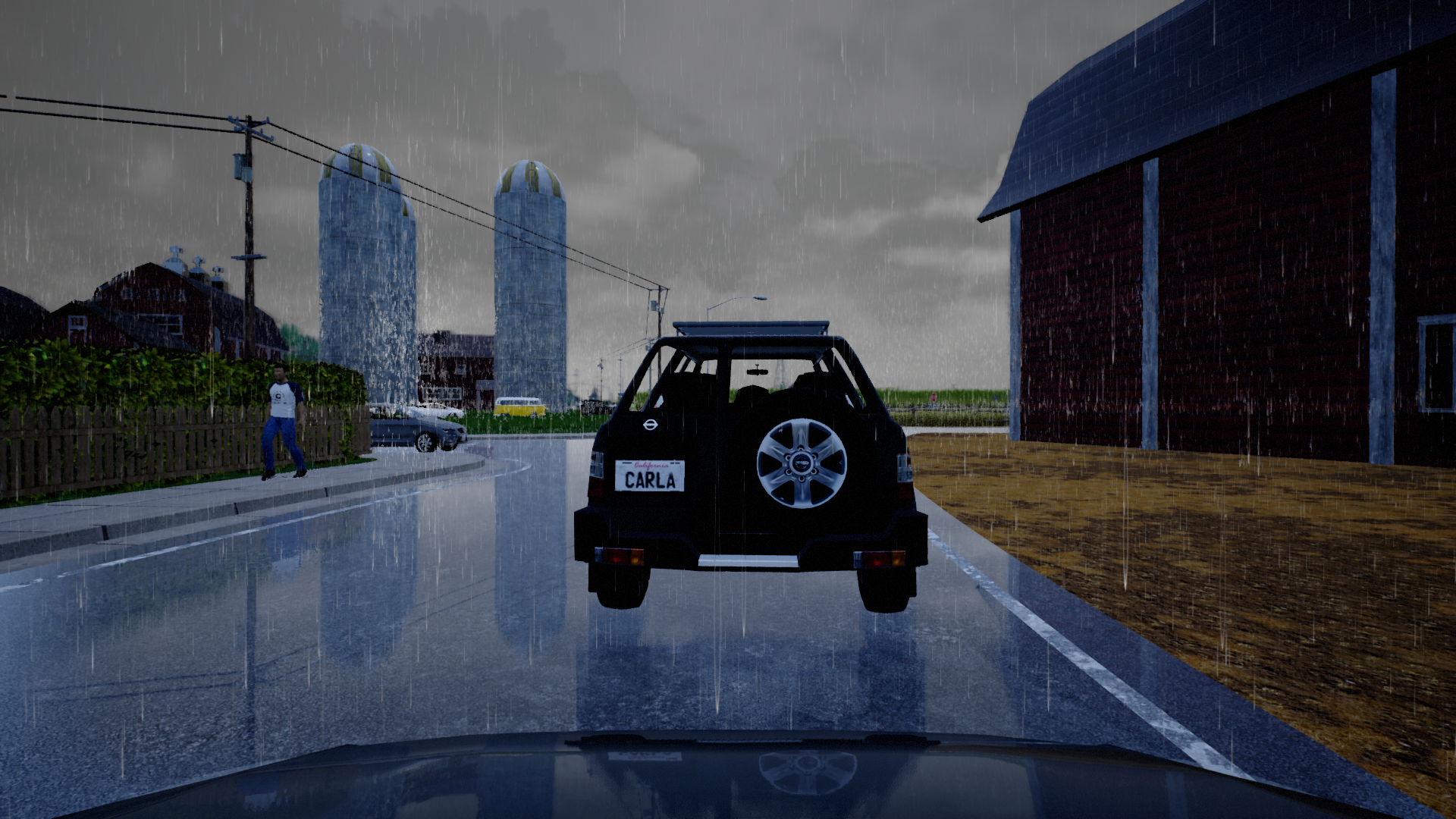
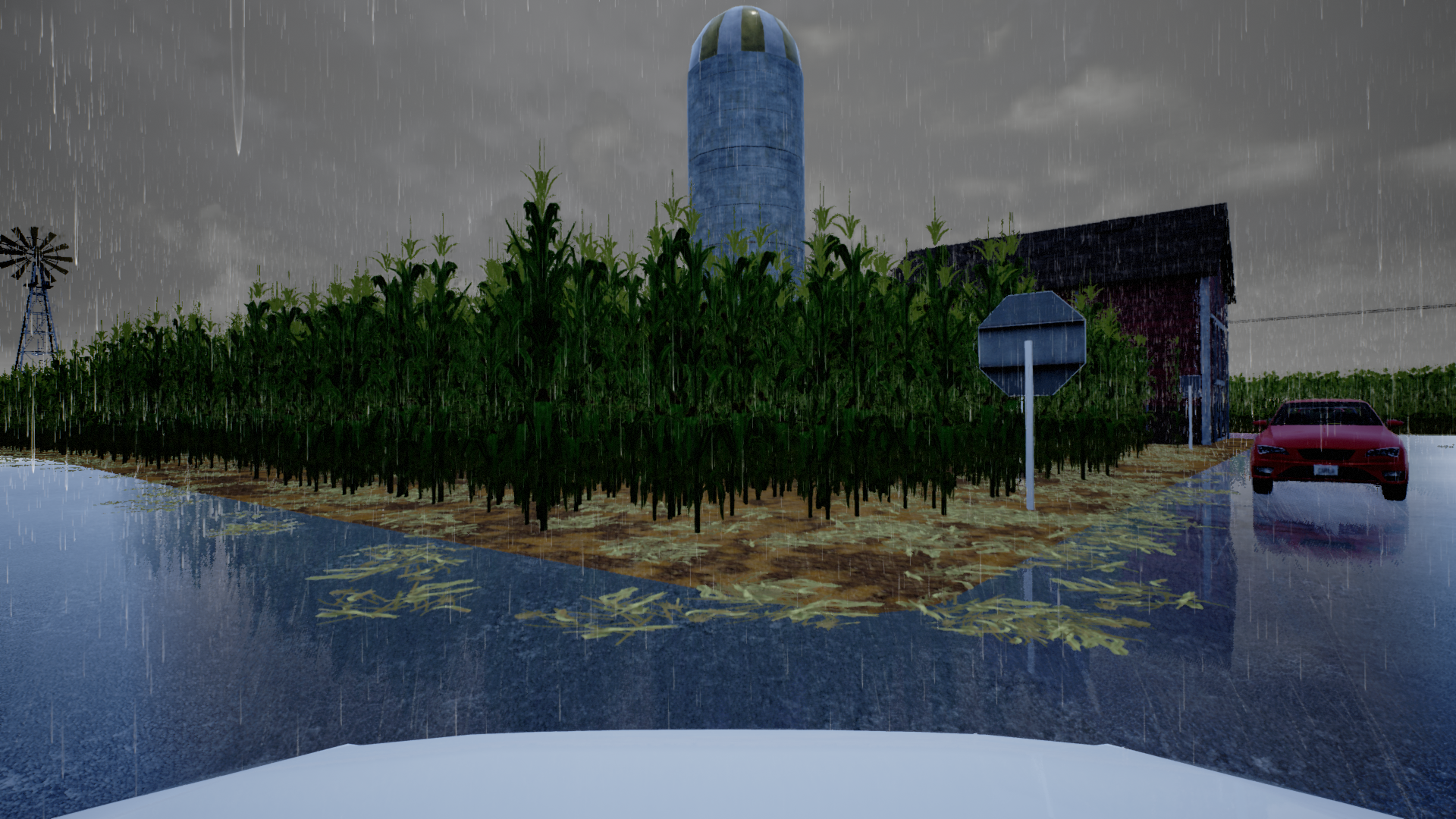
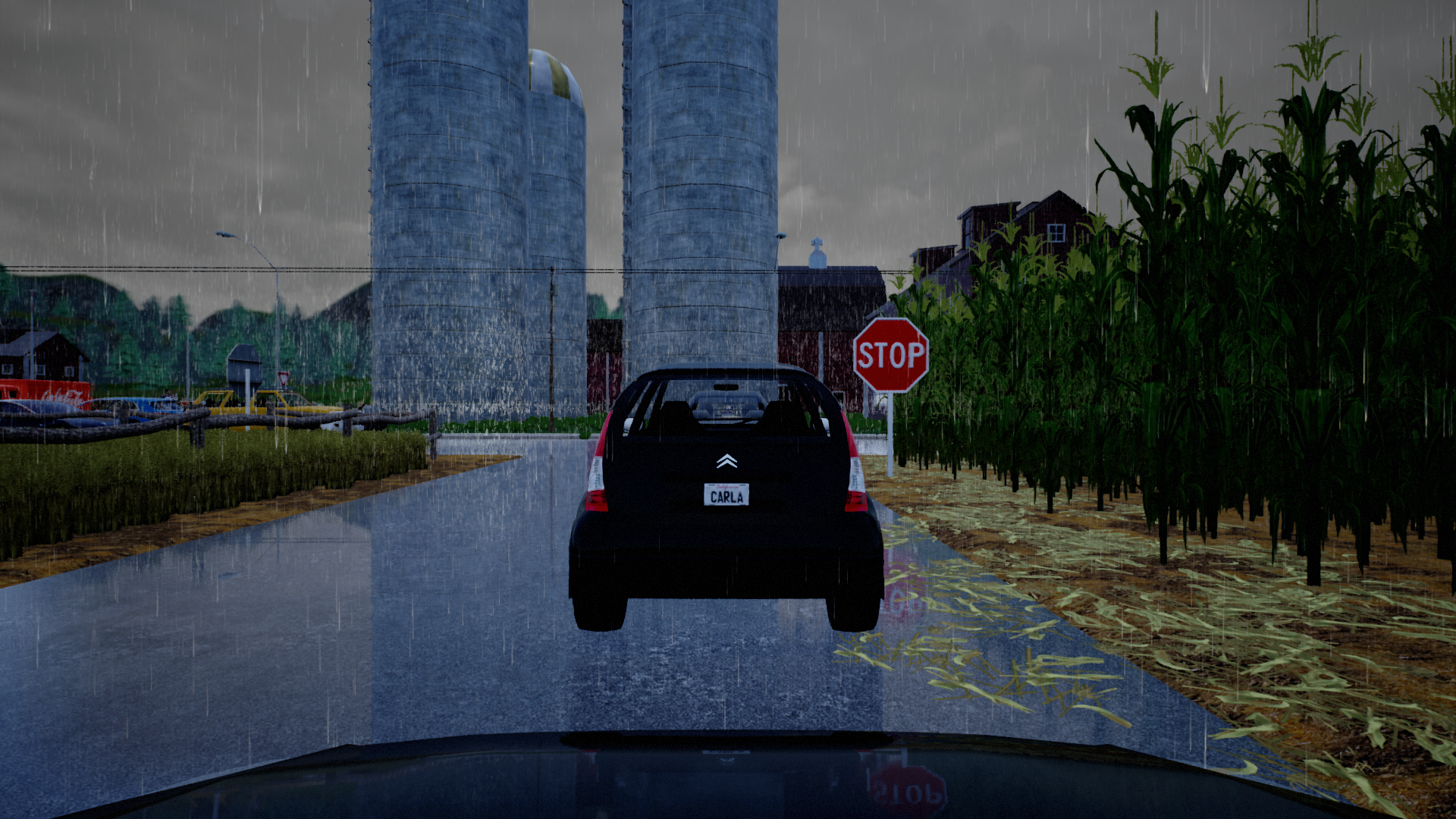
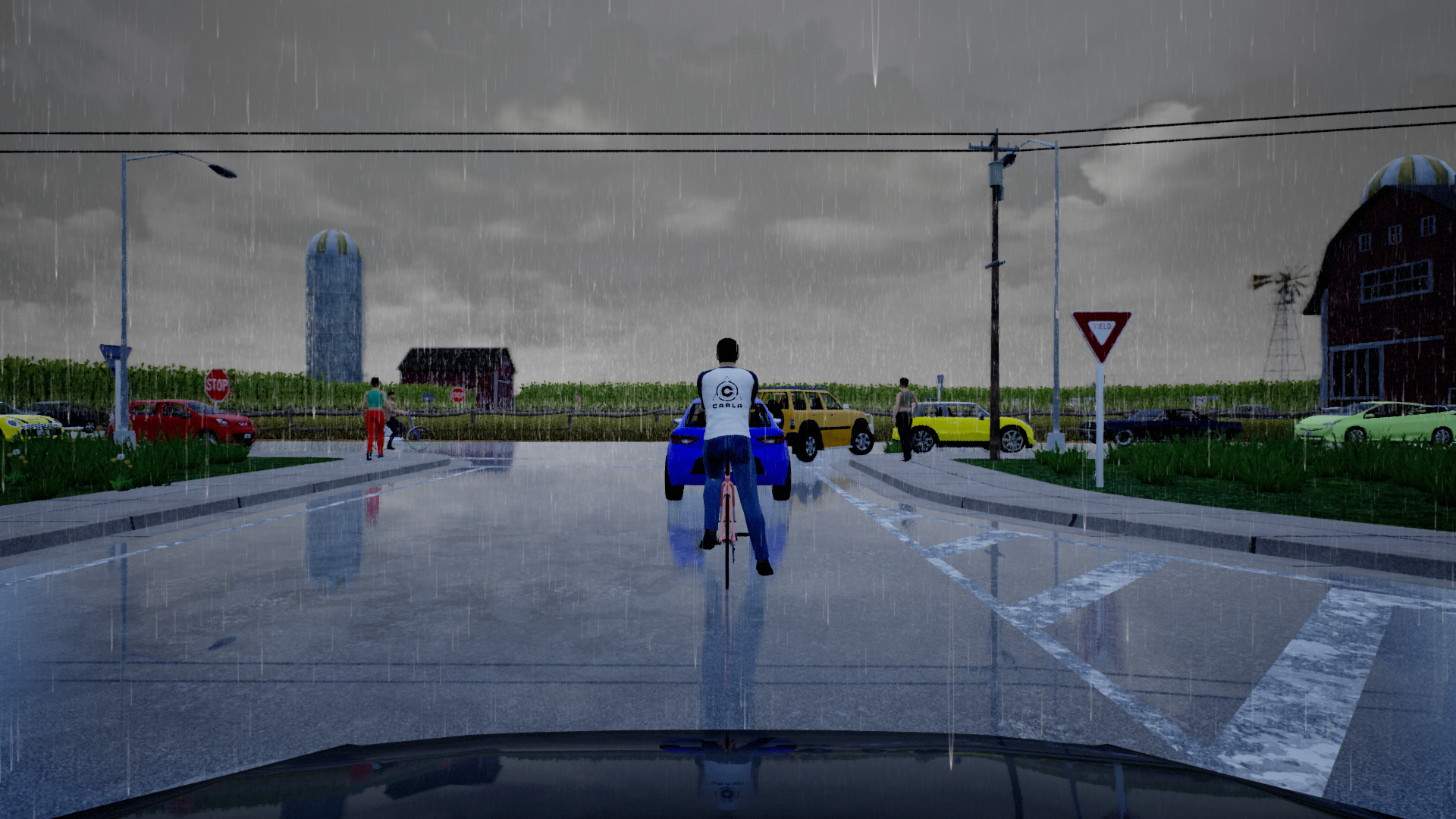
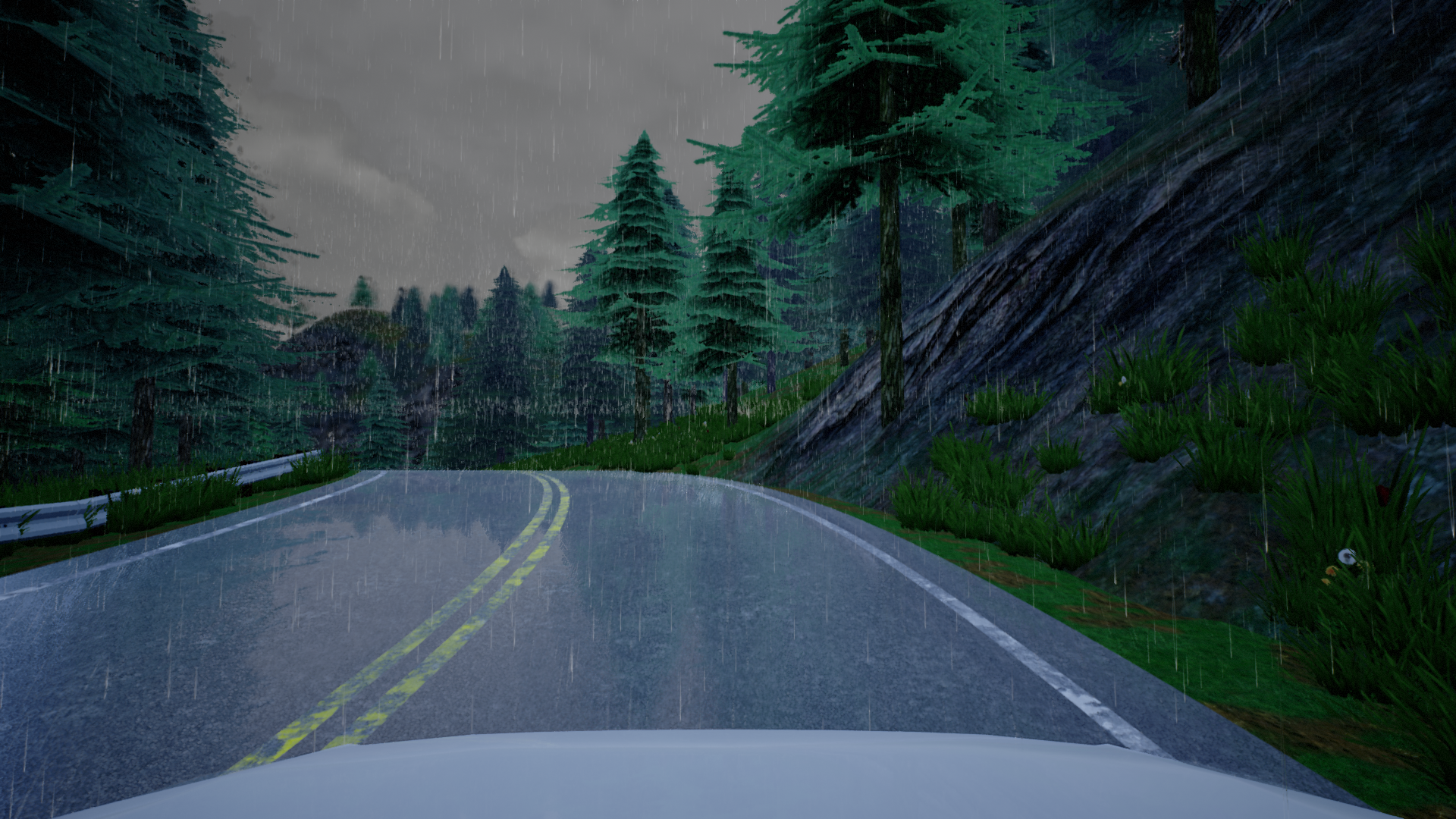
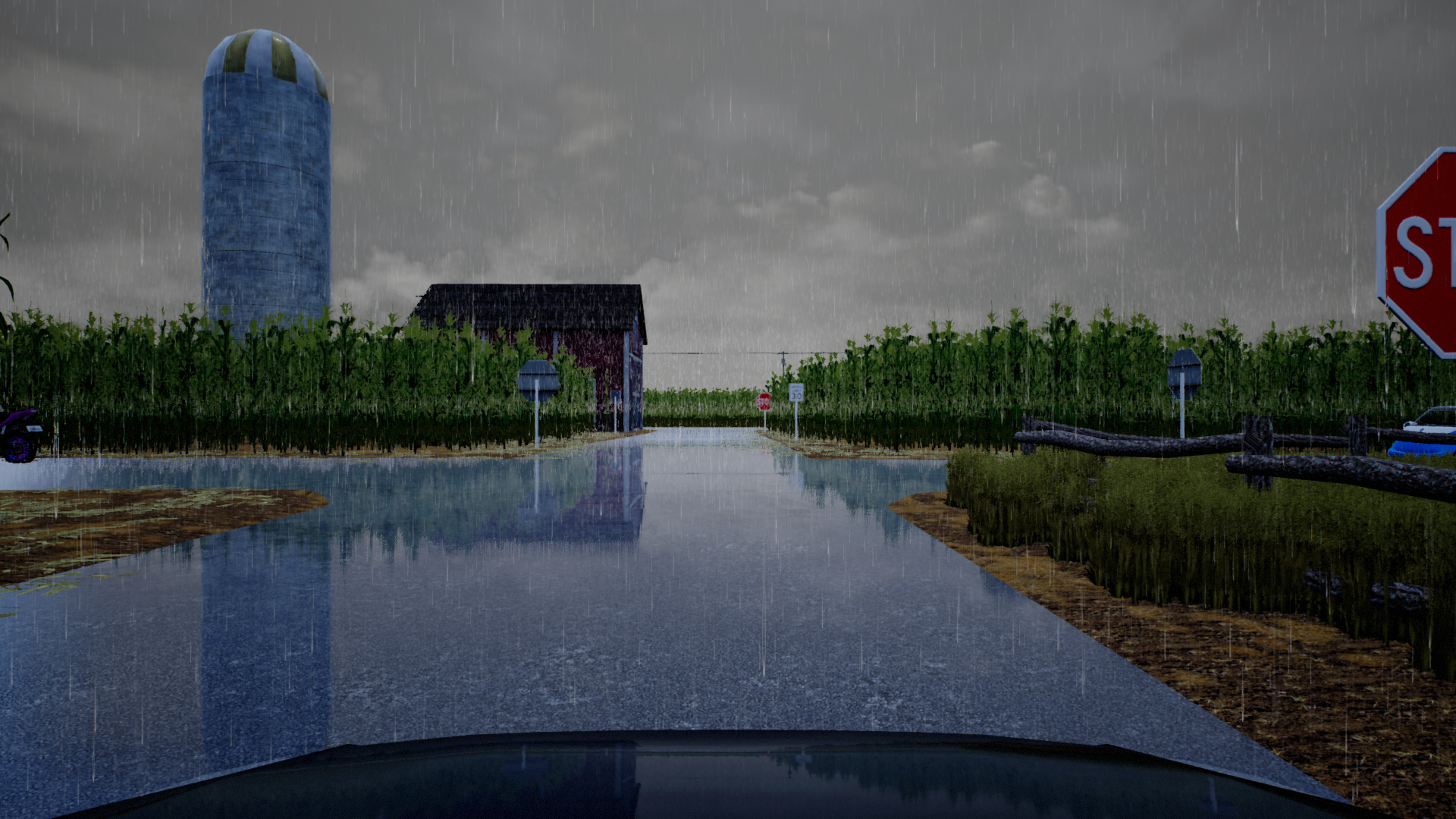
Results
Experiment | Distance Measurement | Performance Evaluation | |||||
---|---|---|---|---|---|---|---|
Euclidean distance |
Cosine distance |
Bhattacharaya distance |
Network | Code Available | mIoU (%) | ||
Source: Town 01, Hard Rain Noon, Audi Target: Town 07, Hard Rain Noon, Audi |
6,4551 | 1,0586 | 0,0426 | without domain adaptation |
DeepLab V2[1] | (soon) | 21,65 |
DeepLab V3+ [2] | (soon) | 14,27 | |||||
PSPNet [3] | (soon) | 14,64 | |||||
PSANet [4] | (soon) | 15,52 | |||||
DeepLab V2 [1] (source=target) |
(soon) | 78,02 | |||||
with domain adaptation |
DADA [5] | (soon) | 36,48 | ||||
ADVENT [6] | (soon) | 39,30 | |||||
CLAN [7] | (soon) | 41,18 | |||||
DISE [8] | (soon) | 46,71 |